Research explores scalable oversight, using weaker LLMs to judge stronger ones, highlighting debate protocols for improved AI supervision and safety.
In the field of AI, ensuring that superhuman AI systems are accurately supervised is critical. Google DeepMind's latest research, "On Scalable Oversight with Weak LLMs Judging Strong LLMs," explores scalable oversight protocols, specifically how weaker language models (LLMs) can effectively judge stronger ones. This study is crucial for business and technology leaders to understand as it directly impacts AI safety and alignment.
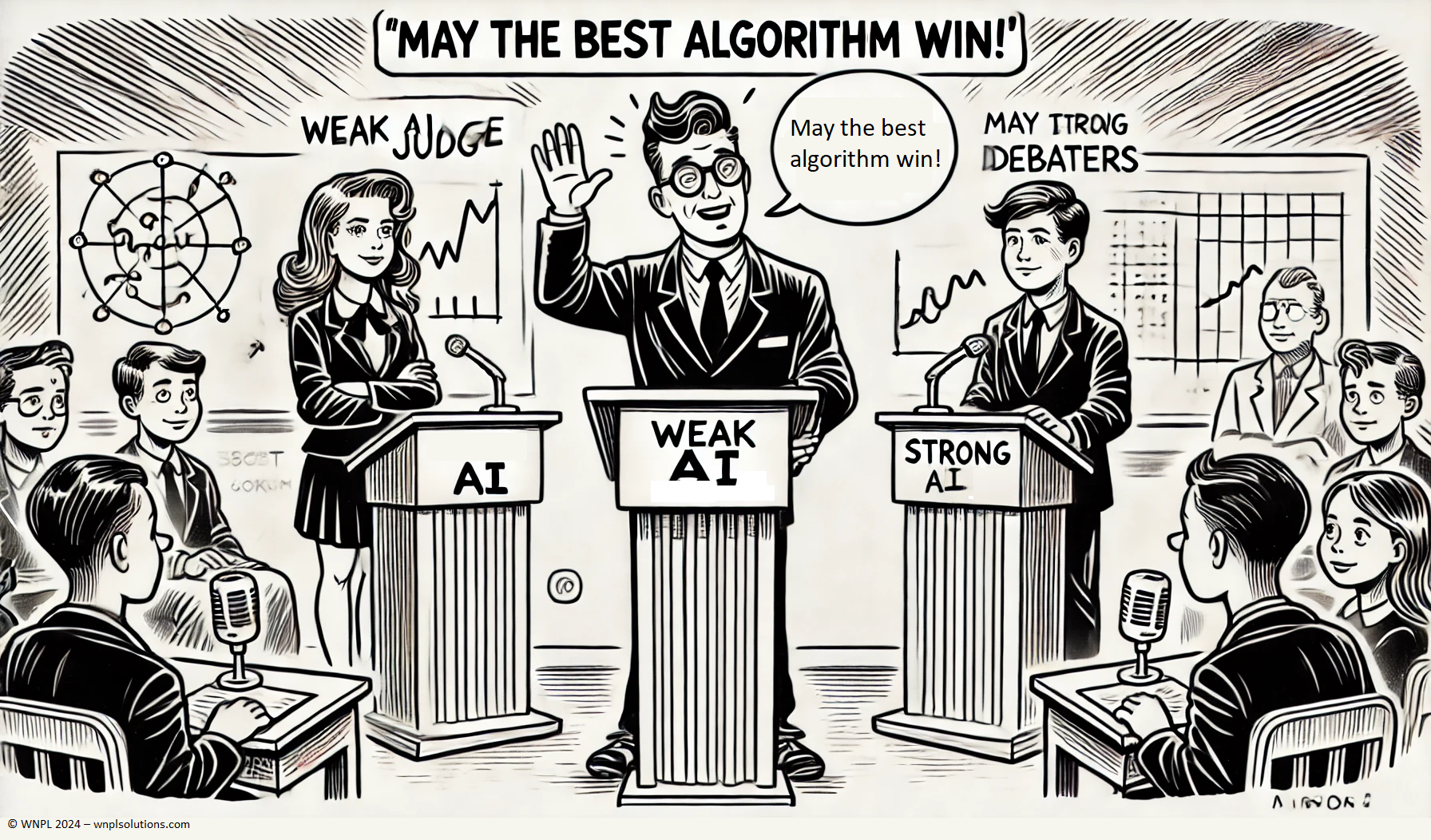
The Need for Scalable Oversight
As AI systems become more advanced, surpassing human experts in various tasks, the traditional human feedback loop becomes inadequate. Scalable oversight aims to enable humans to supervise superhuman AI by leveraging AI's capabilities. This research focuses on different protocols for scalable oversight, namely debate, consultancy, and direct question-answering (QA).
Key Protocols Explored
- Debate: Two AI agents compete to convince a judge, who is a weaker LLM. This setup aims to inform the judge about the merits and flaws of each argument, helping them make a more accurate decision.
- Consultancy: A single AI agent tries to convince a judge by answering questions. The AI is assigned to argue for either the correct or incorrect answer randomly.
- Direct QA: The judge directly answers questions without AI intervention. This serves as a baseline to compare the effectiveness of debate and consultancy.
Methodology and Findings
The study evaluated these protocols across various tasks, including mathematics, coding, logic, and multimodal reasoning, by using large LLMs as both AI agents and judges. Here are some key findings:
- Debate Outperforms Consultancy: In tasks with information asymmetry (where the judge lacks access to the same information as the AI), debate consistently outperformed consultancy. This suggests that when judges are less informed, having AI agents debate helps them make better decisions.
- Mixed Results in Direct QA: When comparing debate to direct QA, results varied based on the task. In tasks with information asymmetry, debate was more effective than direct QA. However, in tasks without such asymmetry, the advantage of debate was less clear.
- Choosing Sides: When AIs were allowed to choose which answer to argue for, judges were less frequently convinced by the wrong answer in debate compared to consultancy. This indicates that debate might be better at filtering out incorrect answers.
Implications for AI Alignment
The research highlights several implications for AI alignment:
- Enhanced Oversight Protocols: Debate shows promise as a scalable oversight protocol, particularly in scenarios where judges have limited information.
- Training and Evaluation: Future work should explore training AI agents using debate as a reward signal, potentially improving the alignment of superhuman AI systems.
- Human-Informed AI Development: Incorporating human judgment, even when using weaker LLMs, can still enhance the oversight of more capable AI systems.
Key Takeaway Points
- Scalable Oversight Importance:
- Essential for accurate supervision of superhuman AI.
- Enhances AI safety by leveraging AI capabilities for human oversight.
- Debate vs. Consultancy:
- Debate protocols outperform consultancy, especially when judges have limited information.
- Helps in making more accurate decisions by presenting arguments from both sides.
- Effectiveness of Direct QA:
- Debate shows mixed results compared to direct QA.
- More effective in tasks with information asymmetry but less clear in others.
- Choosing Argument Sides:
- Allowing AIs to choose their stance in debates results in fewer incorrect answers being accepted.
- Indicates better filtering of incorrect answers through debate.
- Training and Future Research:
- Further research needed on using debate for training AI.
- Potential to improve AI alignment and oversight protocols.
- Business and Technology Implications:
- Understanding scalable oversight is crucial for safe AI integration.
- Implementing effective oversight protocols can enhance AI development and usage.
Advancing Further Research
- Benchmarking Oversight Protocols:
- Provides comprehensive evaluations of different oversight protocols (debate, consultancy, direct QA).
- Establishes benchmarks for future studies to compare and improve upon.
- Highlighting Effective Oversight:
- Demonstrates that debate protocols can enhance judgment accuracy in scenarios with information asymmetry.
- Encourages exploration of debate as a primary method for scalable oversight.
- Identifying Limitations and Areas for Improvement:
- Points out the mixed results of debate vs. direct QA, suggesting areas where debate might need refinement.
- Highlights the need for further studies on diverse tasks and different capability gaps.
- Implications for AI Training:
- Suggests the potential of using debate as a training protocol.
- Encourages research into self-play and other advanced training techniques using weak-to-strong model dynamics.
- Encouraging Real-world Applications:
- Provides a framework for applying scalable oversight in practical AI systems.
- Suggests future work on integrating human judges and real-world datasets to validate findings.
- Promoting Safety and Alignment:
- Emphasizes the importance of scalable oversight for the safety and alignment of superhuman AI systems.
- Supports ongoing research into methods that mitigate risks associated with advanced AI capabilities.
Conclusion
This research underscores the importance of scalable oversight in the safe development of AI systems. By using protocols like debate, where weaker AI models judge stronger ones, we can create more robust and aligned AI systems. For technology leaders, understanding and implementing these findings will be crucial in navigating the future of AI development and ensuring its safe integration into various sectors.
References